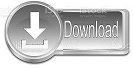
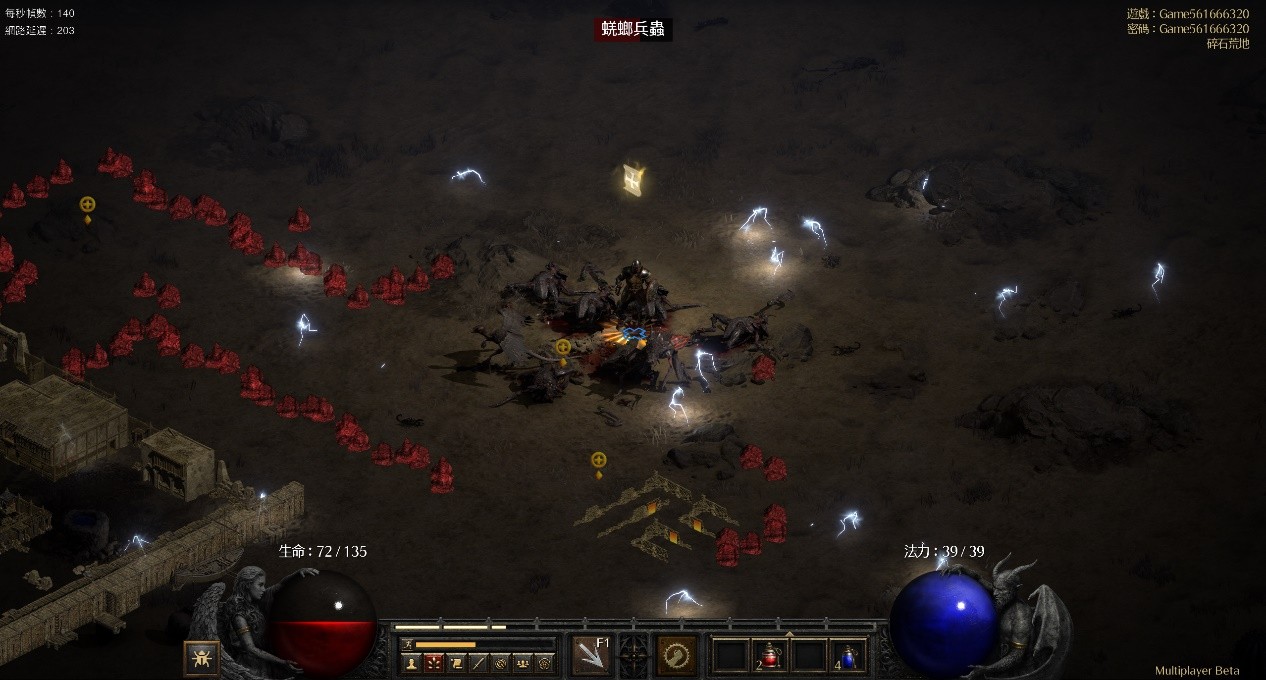
By using the cascade of heterogeneous layers of neural networks to gradually extract increasingly high-level features, they lead to ever-improving neural networks built on their basis. Deep learning models have proven to be useful in increasing the effectiveness of diagnoses of cardiovascular diseases using ECG signals. Such an application would primarily focus on the automatic recognition of patterns that classify various disease entities, a method that is gaining greater importance in medical practice.Īlgorithms known as deep neural networks have become particularly important in the last five years. On the other hand, an alternative method is the application of machine learning methods. In this area, many algorithms are based on three processes: feature extraction, feature selection, and classification. On the one hand, digital signal processing methods mainly include low- or high-pass filters, fast Fourier transform, and wavelet transform. Previous literature on ECG databases has shown a methodological division: signal processing and machine learning. Over the past decade, numerous attempts have been made to identify a 12-lead clinical ECG, largely on the basis of the availability of large, public, open-source ECG data collections. With the rapid development of ECG and, at the same time, an insufficient number of cardiologists, the accurate and automatic diagnosis of ECG signals has become an interesting research topic for many scientists. Incorrectly interpreted ECG results may give rise to incorrect clinical decisions and lead to a threat to human life and health.
#Median xl resolution manual
However, manual interpretation is time consuming and requires skill.

Traditionally, ECG results are manually interpreted by cardiologists based on a set of diagnosis criteria and experience. Īn electrocardiogram (ECG) is a widely used, reliable, noninvasive approach to diagnosing cardiovascular disease. Accurate classification of heart disease types can aid in diagnosis and treatment. However, there are many types of irregular heartbeat. One of the main causes of cardiovascular diseases is cardiac arrhythmia, in which the heartbeat deviates from typical beating patterns. The convolutional network without entropy-based features obtained a slightly less successful result, but had the highest computational efficiency, due to the significantly lower number of neurons.Īccording to publicly available reports, cardiovascular disease remains the leading cause of mortality worldwide. The convolutional network with entropy features obtained the best classification result. The studies were conducted for 2, 5, and 20 classes of disease entities. The dataset was divided into training, validation, and test sets in proportions of 70%, 15%, and 15%, respectively. Three neural network architectures were proposed: the first based on the convolutional network, the second on SincNet, and the third on the convolutional network, but with additional entropy-based features. The research was carried out on the data contained in a PTB-XL database. In this work, a deep neural network was developed for the automatic classification of primary ECG signals.


The main field of work in this area is classification, which is increasingly supported by machine learning-based algorithms. The analysis and processing of ECG signals are a key approach in the diagnosis of cardiovascular diseases.
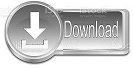